How is your
website's SEO?
Use our free tool to get your score calculated in under 60 seconds
How to Prevent Fraud Detection in E-commerce Using AI
How is your
website's SEO?
Use our free tool to get your score calculated in under 60 seconds
By Ramsha Irfan on May 25, 2023
-
Share on
-
Share on
-
Share on
AI offers significant advantages in fraud detection by leveraging advanced algorithms and machine learning techniques. It can quickly analyze vast amounts of data, identify patterns, and detect anomalies, enabling proactive fraud prevention. AI-powered fraud detection systems continuously learn and adapt based on evolving fraud patterns, enhancing their accuracy over time.
By employing AI in fraud detection, e-commerce businesses can detect fraudulent activities early, reducing financial losses and protecting customers. To identify potential fraud indicators, AI algorithms can analyze multiple data points, including transactional data, user behavior, device information, and historical patterns.
Furthermore, AI can help automate fraud detection, enabling businesses to scale their operations without compromising accuracy. It enhances efficiency by reducing false positives and negatives, optimizing resource allocation, and minimizing manual intervention.
The Growing Need for Fraud Detection in E-commerce
Fraud has never been a novel concept, yet the prevalence of cash-free transactions is increasing the trend for E-commerce fraud. At this time, when the globe is turning away from in-store shopping, it is especially clear.
People are forced to make additional online purchases to keep safe during the COVID-19 quarantine or because they can't find the items they need at the local closed stores.
Since 2020, as online sales have risen quickly, e-commerce fraud has changed and grown. According to estimates, online payment theft may cost e-commerce firms $200 billion by 2024. Even if there has been a rise in e-commerce fraud, these attacks are becoming more complex thanks to criminal actors.
Common Fraud Schemes in E-commerce
Account takeover
It involves unauthorized access to a customer's account, allowing fraudsters to make fraudulent transactions or gather personal information.
Identity theft
Identity theft occurs when fraudsters use stolen personal information to impersonate legitimate customers and make unauthorized transactions.
Payment fraud
Payment fraud involves fraudulent transactions using stolen credit card information, fake payment methods, or chargebacks.
Return fraud
Return fraud occurs when fraudsters exploit the return policies of e-commerce businesses, returning stolen or counterfeit items for a refund.
Traditional Fraud Detection Methods
Manual review: Human analysts carefully examine transactions to identify suspicious patterns or anomalies that may indicate fraudulent activity.
Rule-based systems: Rule-based systems utilize predefined rules and criteria to flag potentially fraudulent activities based on specific patterns or conditions, helping automate detection.
Limitations of Traditional Methods:
- High false positives and negatives
- Time-consuming and labor-intensive
- Inability to adapt to evolving fraud patterns
AI Techniques for Fraud Detection
Supervised Learning
Supervised learning involves training AI models using labeled data to classify transactions as either fraudulent or legitimate based on known patterns.
Unsupervised Learning
Unsupervised learning is an AI technique used in fraud detection that focuses on identifying anomalies in data without relying on labeled examples. It enables the system to detect patterns and irregularities that may indicate fraudulent behavior, even without prior knowledge of specific fraud instances.
Deep Learning
Deep learning is a type of machine learning that processes and analyses complicated data structures using artificial neural networks. In fraud detection, deep learning algorithms may automatically create hierarchical data representations, allowing them to find subtle patterns and make accurate predictions even in highly complex and unstructured datasets.
Natural Language Processing Techniques
Natural language processing techniques allow AI systems to analyze and comprehend textual data such as customer reviews, social media postings, and chat logs to detect possible fraud indications.
Implementing AI for Fraud Detection in E-commerce
Data Collection and Preparation
Collecting relevant data, including transactional data, user behavior, device information, and historical patterns, and preparing it for analysis is crucial in implementing AI for fraud detection.
Model Training and Validation
AI models must be trained on historical data to learn patterns and detect fraud accurately. Validation ensures the model's effectiveness before deployment.
Developing a Virtual Reality Application for an E-Commerce Store
Developing a virtual reality (VR) application for an e-commerce store is another method of integrating AI for fraud detection in e-commerce.
Businesses may develop realistic virtual shopping experiences that improve consumer engagement and lower the risk of fraud by offering a secure environment for transactions by utilizing AI and VR technologies.
Integration with Existing Systems
Integrating AI-based fraud detection systems with existing e-commerce platforms, payment gateways, and fraud management tools is essential for seamless operations.
Continuous Monitoring and Improvement
Fraud detection models should be continuously monitored to adapt to new fraud techniques and evolving patterns. Regular updates and improvements are necessary to maintain high accuracy rates.
How Does AI Help Fraud Detection in Banks?
AI plays a crucial role, helps to reduce fraud detection in banks as fraud techniques become increasingly sophisticated, necessitating advanced detection methods.
Fraudsters continuously evolve their tactics, making traditional rule-based systems less effective. AI offers a proactive approach to detecting fraud by leveraging advanced algorithms and machine learning techniques.
AI techniques such as machine learning and deep learning are used in fraud detection.
- Machine learning algorithms analyze historical data to identify patterns and create models for fraud detection.
- With its neural networks, deep learning excels at detecting complex patterns in large datasets.
Examples of AI Applications in Banks
AI is used in banks for various fraud detection purposes, such as anomaly detection and transaction monitoring
Anomaly detection algorithms identify unusual patterns or behaviors that may indicate fraud, while transaction monitoring systems analyze transactions in real-time to identify suspicious activities.
Benefits of Using AI in Fraud Detection
Improved Accuracy
AI-powered fraud detection systems leverage advanced algorithms and machine learning techniques to achieve high accuracy rates in identifying fraudulent activities.
Faster Detection
Real-time analysis of transactions and user behavior enables AI systems to detect fraud promptly, minimizing financial losses.
Reduced False Positives
AI techniques help reduce false positive rates by accurately identifying legitimate transactions, enhancing the efficiency of fraud detection processes.
Enhanced Customer Experience
By minimizing false positives and negatives, AI-based fraud detection systems provide a better customer experience by reducing unnecessary transaction declines and ensuring security.
Challenges and Limitations of AI in Fraud Detection
Data Privacy Concerns
The use of AI in fraud detection requires access to sensitive customer data, raising concerns regarding data privacy and security.
High Implementation Costs Implementing
AI-based fraud detection systems can be costly, including investments in infrastructure, data acquisition, and hiring skilled professionals.
AI technologies require skilled data analysis, machine learning, and fraud detection professionals to implement and maintain fraud prevention systems effectively.
Adapting to New Fraud Techniques
Fraudsters continuously evolve their techniques, necessitating ongoing updates and improvements to AI models to stay ahead.
Will AI Impact Business in the Future?
This question, "Will AI Impact Business in the Future?" is rising!
Artificial intelligence (AI) may automate tedious and mundane operations, freeing up human resources for more strategic and innovative projects. It can analyze enormous amounts of data to offer insightful information that helps firms make wise decisions and achieve a competitive advantage.
- In healthcare, AI assists in medical diagnoses and drug discovery.
- In finance, AI powers algorithmic trading and fraud detection systems.
- In manufacturing, AI optimizes production processes and predictive maintenance.
While AI offers numerous benefits, concerns about job displacement arise. Businesses must invest in upskilling and reskilling their workforce to adapt to the changing landscape.
Responsible AI implementation is essential to address bias, privacy, and transparency issues. Businesses must prioritize ethical considerations to ensure AI benefits society as a whole.
Conclusion
Implementing AI in fraud detection for e-commerce brings significant benefits to businesses. By leveraging advanced algorithms, machine learning, and data analysis techniques, AI enhances accuracy, speeds up detection, reduces false positives, and improves the overall customer experience. As fraud techniques evolve, AI plays a crucial role in safeguarding e-commerce operations and fostering trust between businesses and customers.
Frequently Asked Questions
Can AI help reduce false positives in fraud detection?
Yes, AI algorithms can continuously learn and adapt to new fraud patterns, leading to more accurate and precise identification of fraudulent activities while minimizing false positives.
Is AI capable of detecting evolving and sophisticated fraud techniques?
Yes, AI can adapt to changing fraud techniques by leveraging advanced techniques like deep learning, neural networks, and anomaly detection, enabling the detection of even the most sophisticated fraud attempts.
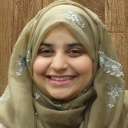
Ramsha Irfan
Ramsha is a talented writer known for top-quality content on trending topics. Her excellence in research enables her to add value to businesses by driving online traffic with engaging and persuasive content.